Right now there is a lot of hype around LLMs for good reasons : they can provide great help and their capacity to convince you, whether the output is right or not is spectacular. It reminds me of a lot of politicians.
Just to bring back the theory behind LLMs, it’s about making statistics on a large volume of text so you know what will happen next in the phrase. It’s using bits of words called tokens, but I will simplify here : for example “I’m going to the garage to pick my …” the machine knows there’s a high probability the next word would be car. Obviously it’s just statistics, because it could be a motorcycle, or a tool, or a dog. So the tech that was at the beginning just helping you complete your SMS was trained with an awful lot of texts. Find here a nice article if you want to dig in the details, it’s not too technical and a very good starting point.
The problem with a system based on statistics is that it’s predictable… up to an extent. I love statistics because in a mathematical system they bring flexibility : you don’t know exactly the value, but you know it’s within a range, for example, body weight for humans :
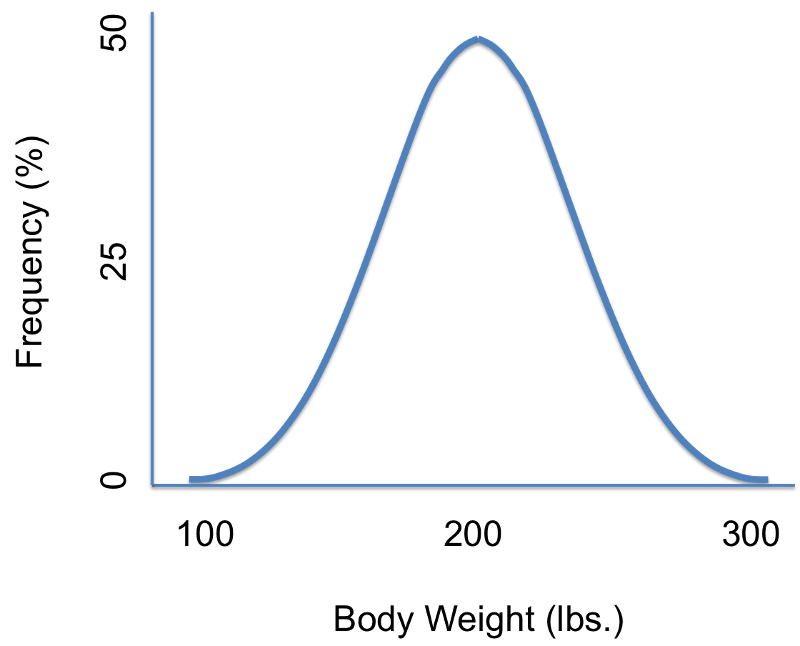
In a mecanical system, flexibility is brought by the right choice of materials and shapes. Putting together an efficient system is very complex, take the example of a bike frame :
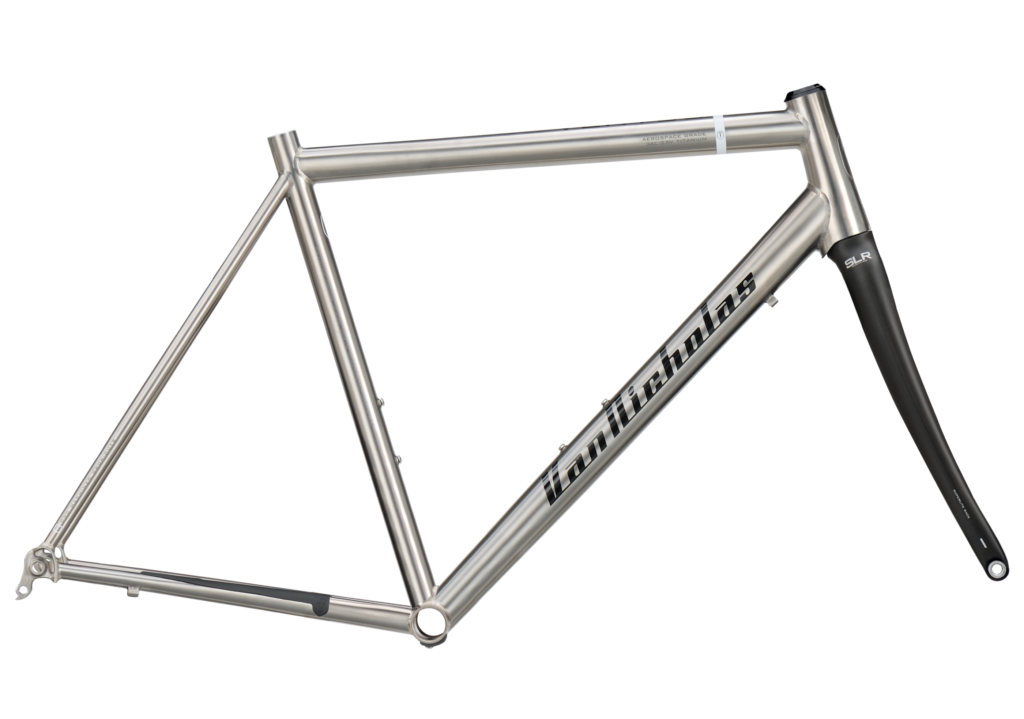
Bicycles are amazing : they are very efficient at transforming calories into movement. But it’s not easy to build a good bicycle : it takes to have some very rigid parts in some places to maximize the energy conversion, some flexibility in some other places for comfort and bounce capabilities when needed. It’s a very complex mechanical system, and those here who know how much a bicycle can change by swapping the wheels for better ones know what I’m talking about.
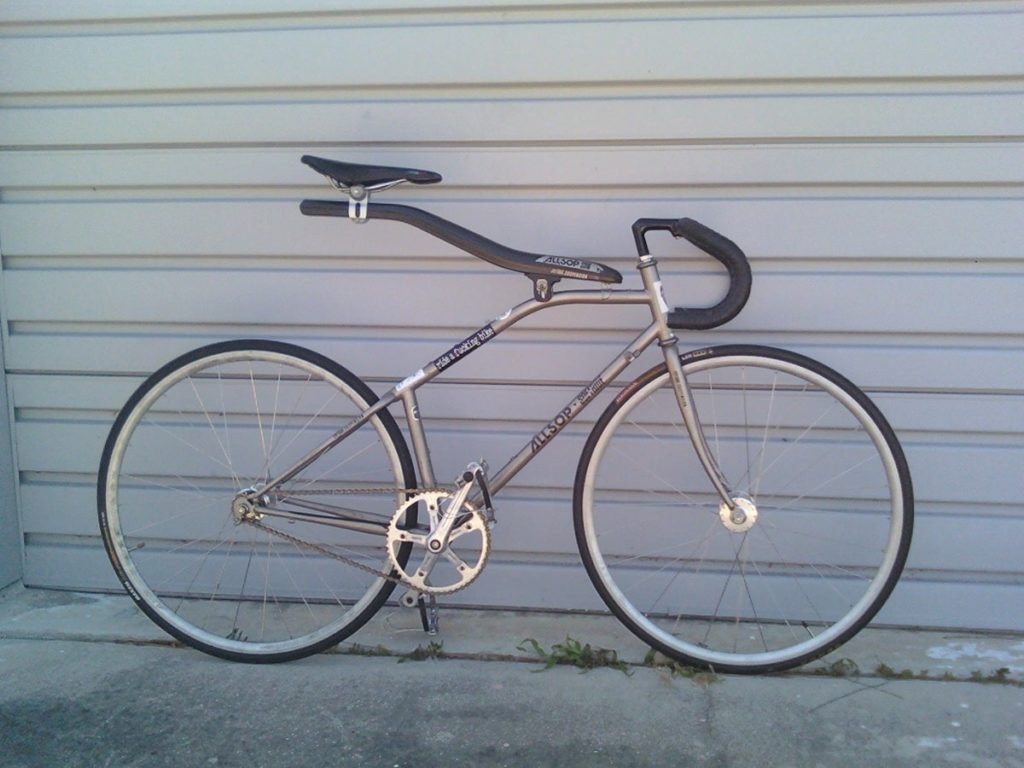
Which brings me to my analogy : information systems are structures with moving parts. You want to have to have a robust system that gives you predictable results, and that is nice to use. So you build a system with a solid organization and processes, but it generates a lot of discomfort for users, because it’s too stiff, like a bad aluminum frame. In most cases, companies put processes in some places, but at the end they still lack the data to have a nice performance overview. One of the main reasons pointed by the last RAND report about AI projects, telling that a whopping 80% of them fail (!!), is that “many AI projects fail because the organization lacks the necessary data to adequately train an effective AI model.”
The opportunity with LLM based AI is that it can bring some little bits of that flexibility to the system so people can actually use it : chatbot instead of a User Manual that nobody wants to read, automations for document processing, meeting summaries… it can be kept very simple, cheap and bring a lot of value to users.
The funny part is that LLM adoption is already happening as a lot of people are already using GPTs for a lot of things in their daily tasks, which can bring confidentiality problems, but it’s certainly a bottom up trend. And pretty much the same as Gmail changed the perception of enterprise email and iPhones changed the way people interact with software, we can expect this experiment to have an influence over the future of enterprise information systems.
Back on the bike!
Cedric